Challenges and Solutions in Stochastic Reservoir Modelling (EET 12)
Geostatistics, Machine Learning, Uncertainty Prediction
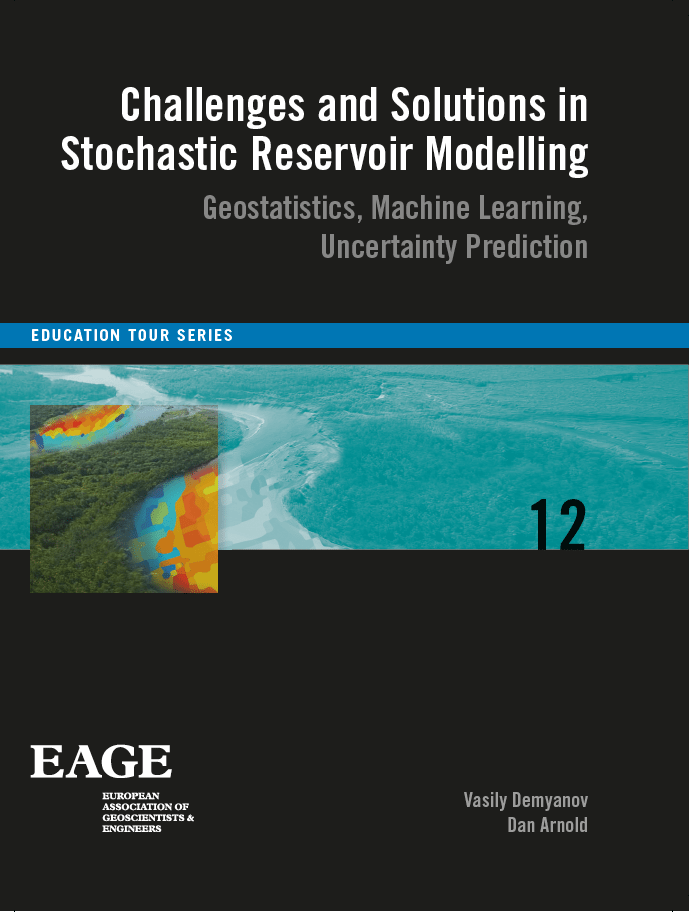
- EPUB
Many advances in stochastic reservoir modelling have been introduced in the past decade. Novel method of data integration and more accurate representation of geology have been developed with the advances in spatial statistics. However, integrated approach for predictive reservoir modelling still attracts continuous effort to manage reservoir decisions under uncertainty and make better use of the increasing amounts of data and domain knowledge accumulated in the field.
Many solutions to these challenges lie in the cross-disciplinary vision, where modern rigour of computer science and statistics brought together with core geological and engineering domain expertise and basic physical conceptual thinking.
This book aims to bridge across different fields — geostatistics, machine learning, and Bayesian statistics — to demonstrate the common grounds in solving challenging problems of uncertainty quantification, geological realism, and data integration in reservoir prediction. It presents an overview of key concepts and some of the basic and more advanced algorithms for reservoir modelling and uncertainty quantification. This book includes several practical examples to reinforce the learning outcomes. A tutorial on decision making under uncertainty provides a practical way to apply integrated thinking to a real field dataset.